Training Helpful, Honest, and Harmless AI is a relatively new field that is rapidly evolving to better suit business needs. One such advancement is the use of synthetic data to supplement real data, which can be more cost-effective and avoid privacy concerns.
In this blog series, we've spoken with experts at Invisible to share insights, today's article will focus on some of the challenges in generating and validating the quality of synthetic data.
Recap
In our first post, we explained what synthetic data is, how it is produced, and why businesses should embrace it. In our last post, we reviewed two main approaches to generating synthetic data and how it benefits businesses.
The challenge of generating usable synthetic data
Creating high-quality synthetic data is challenging. It's not just about producing large quantities of data; the data must be diverse and accurate enough to be genuinely useful for training models.
Hurdles include:
- Diversity vs. Consistency: The data needs to cover a wide range of scenarios to be useful, but it also needs to be consistent and accurate. Balancing these two aspects is difficult.
- Human Effort: Curating and filtering synthetic data to ensure it meets quality standards often requires significant human intervention, making the process labor-intensive and costly.
- Risk of Model Collapse: Training models on synthetic data that is too similar can lead to "model collapse," where models start imitating each other and lose their ability to perform genuinely useful tasks.
Progress towards usable synthetic data
There’s been significant recent progress in methodologies for usable synthetic data development, including most recently from Microsoft’s AgentInstruct framework for Orca3.
AgentInstruct uses a small number of raw text documents and code files as seeds to generate large amounts of useful training data, in this case approximately 22 million instruction sets, which can then be used to train the main model.
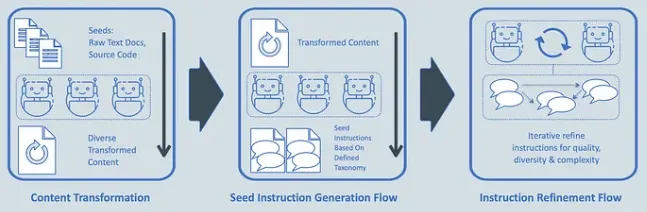
Validating quality
Curtis Macdonald, AI Product Manager at Invisible, notes that “Models will continue to improve both as size and training data increases, but while compute power continues to grow, synthetic data is one of the few scalable sources still untapped for new data to feed into these models.”
But how does a company feel confident in the data that was synthetically generated? Macdonald’s view is to keep humans in the loop: “Human curation and filtering of synthetic data remains a critically important step in validating the quality of data.”
Ready to talk about how to use synthetic data to improve your business?
At Invisible, we consult with businesses spanning industries like Finserv, Healthcare, Retail, Hospitality, and more on their AI strategies. Visit our Get Started page to set up a conversation.